Why the trade-off between sample size and precision of supervision matters and how can we save money?
- Federico Nutarelli
- Jan 19, 2022
- 1 min read
Updated: Dec 27, 2023
The increasing cost of data is posing a significant challenge for institutions, with rising prices potentially restricting access to these vital resources. This situation is emphasized by McKinsey, which points out the growing need for tech companies to manage their internal costs in an era of inflation. Meanwhile, the Dallas Federal Reserve has observed that high inflation is hitting low-income groups the hardest, a trend that could mirror the difficulties institutions face when budget constraints limit their ability to purchase expensive datasets.
Data costs are raised also by the need for users to highly sophisticated protections to avoid breaches which are often sold by the provider of the data with the data themselves. These insights underscore the urgent need for strategic and cost-effective data management practices in today's economically pressured environment.
In other words, the key question is: how can institutions save money and time spend in managing huge amount of data?
In a recent work we contribute to the topic. Namely, that of optimizing the acquisition costs (time and money) of big data to create novel opportunities for data analysts (Sivarajah et al., 2017).
Is having "many but bad" examples always worse –in terms of minimization of the generalization error– than having "few but good" examples in a balanced fixed effects context with correlated errors?
Well, not surprisingly, it turns out that ......... it depends. Go and check out our article published in Machine Learning (Springer) if you are curious. Below a little spoiler on the results:
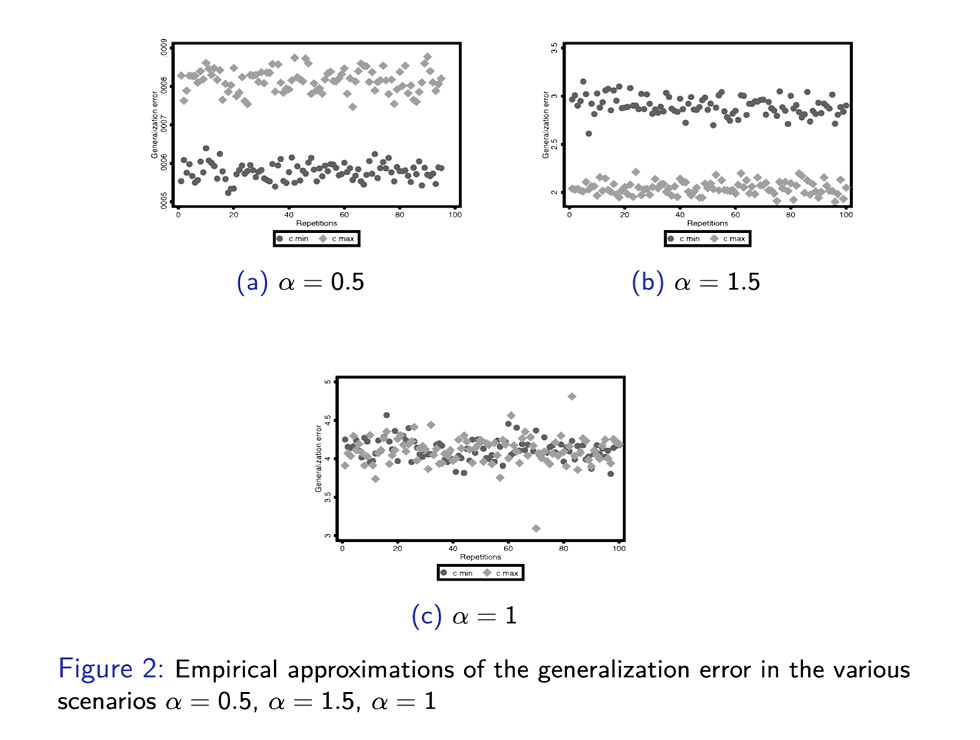
Comments