Which metropolitan city will innovate next?
- Federico Nutarelli
- Sep 12, 2023
- 2 min read
Updated: Jan 9, 2024
Nowadays we hear a lot about smart cities. But what are they in essence and what is the term "smart" referring to? Here is a quick video which answers these questions and which is key to understand the importance of data handling in smart cities:
Thus, in the realm of smart cities, data and connectivity are the cornerstones of innovation. Recognizing which cities best harness these assets is becoming increasingly important. As connectivity expands into broader metropolitan areas, pinpointing the next hub of urban innovation is key. Such foresight can catalyze the development of interconnected, intelligent metropolitan spaces, paving the way for smarter living and efficient urban management.
In the work that this post id referring to, we compare matrix completion - a machine-learning technique often employed in the context of recommender systems - with a benchmark machine-learning model (random forest) to predict the future competitiveness of global cities in several technological areas based on a new patent dataset. Louvain community detection is employed as a pre-processing step to find similar innovation profiles of cities, facilitating the application of matrix completion and random forest:
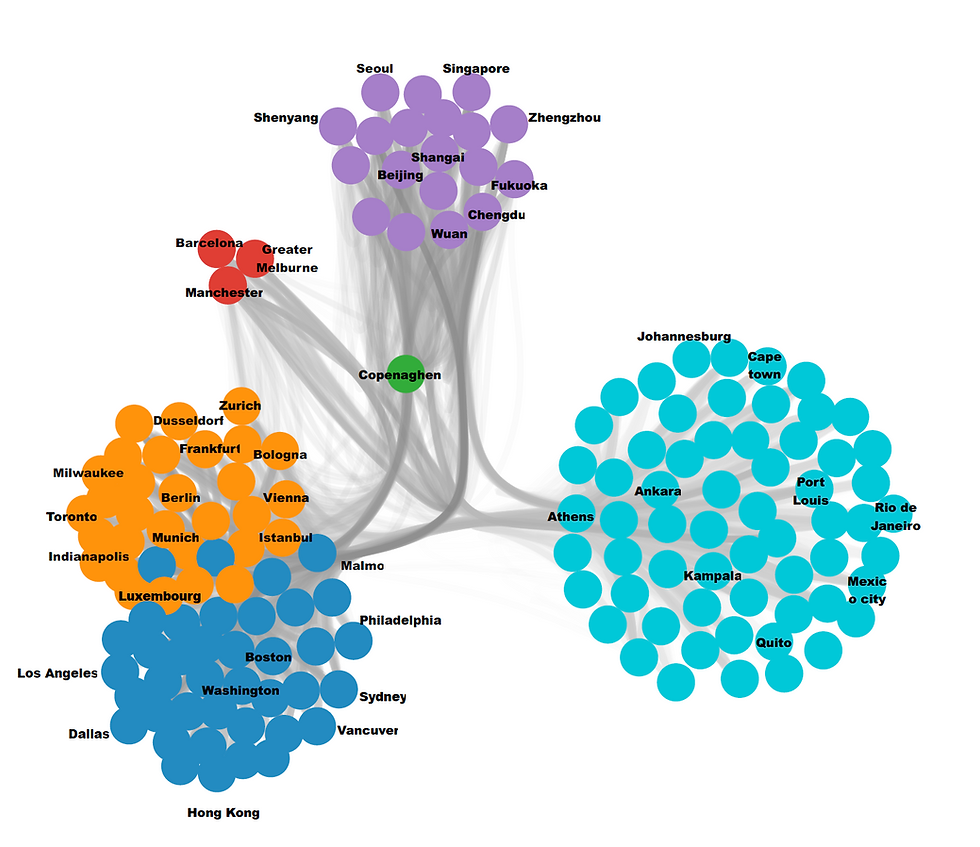
You might be curious about why there's a growing interest in using machine learning for forecasting technology trends, especially in metropolitan cities. You might wonder, don't we already have methods like Hidalgo's Product Space that provide a framework for predicting which patents cities will adopt next, or which ones will be the pioneers in innovation? Yes, we do have such approaches, but they mainly rely on network structures. This means they use adjacency matrices to connect technologies in the product space.
So, what's the issue with that? The key challenge is that these methods might not fully capture higher-order correlations. To explain further, let’s take the example of patenting an electric car. Successfully developing an electric car patent might require expertise not just in one field, but in multiple areas like electronics and mechanics. This scenario shows a complex interplay among these different fields. Traditional methods might miss these nuanced, multi-dimensional relationships, which are crucial for understanding and predicting technological advancements.
Results demonstrate that matrix completion reaches superior prediction performance as compared to the benchmark model. Our contribution shows how supervised machine learning can support local innovation and development policies.
Unfortunately I cannot show you too much as the paper is still a work in progress....however below are some interesting results that may catch your attention if you read until here:
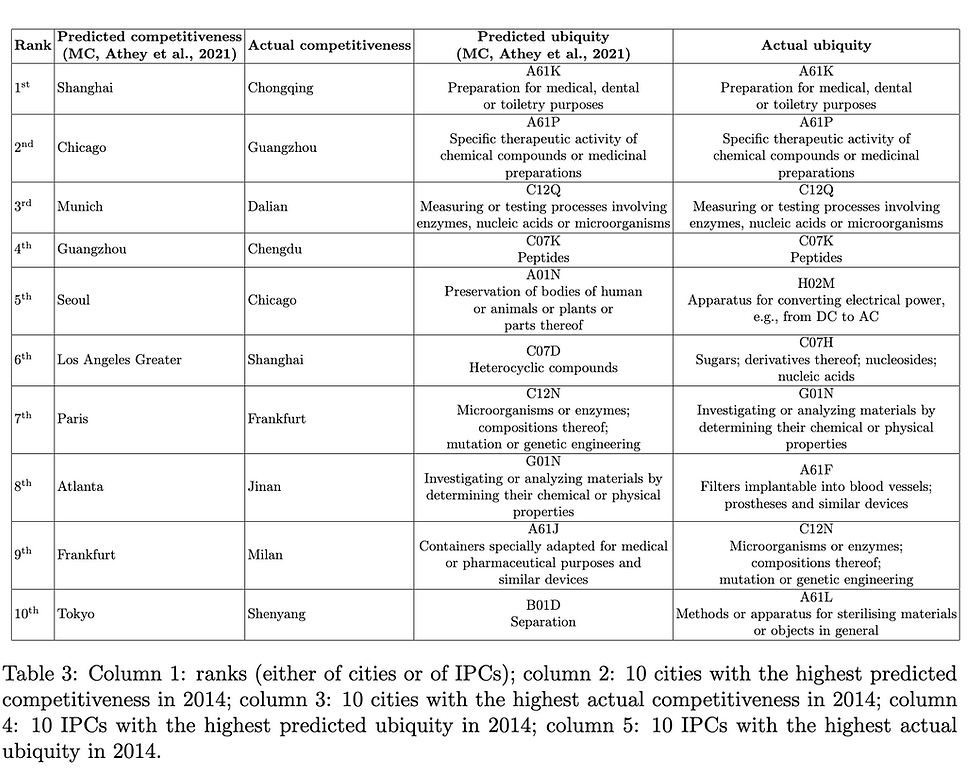
Commenti